Advantages of Thermal Images over Visible Images for Facial Analysis
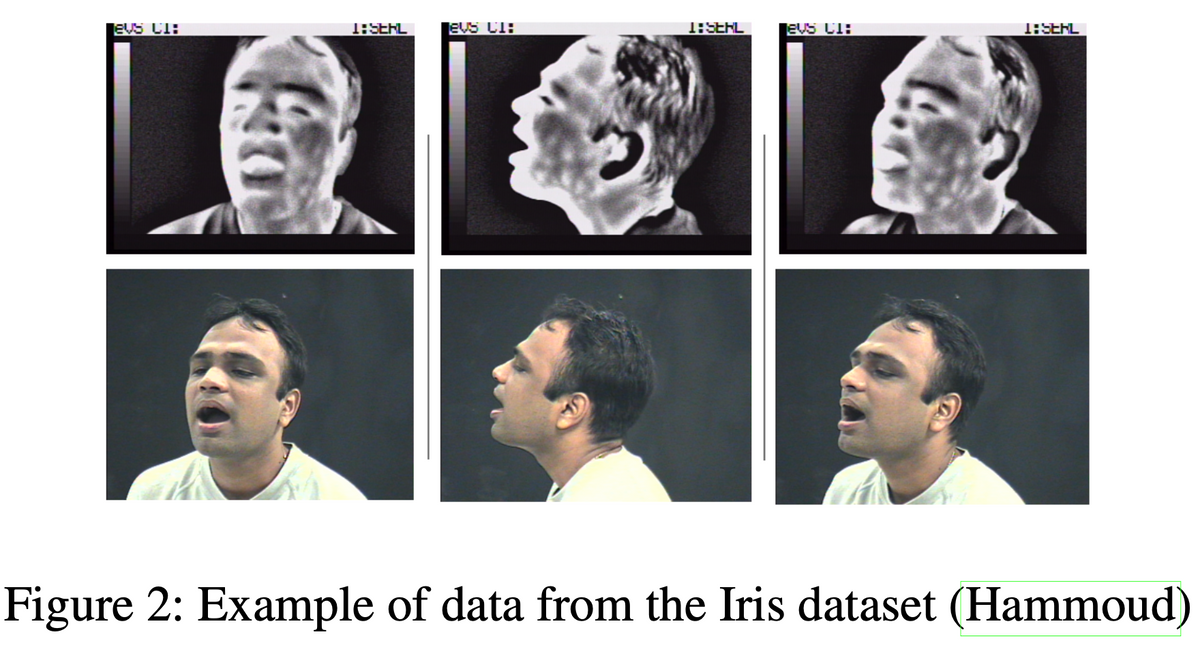
Below is an excerpt from our paper Ordun, Catherine, Edward Raff, and Sanjay Purushotham. "The Use of AI for Thermal Emotion Recognition: A Review of Problems and Limitations in Standard Design and Data." arXiv preprint arXiv:2009.10589 (2020) for AAAI Government and Public Sector Symposium 2020. YouTube video here.
When the public sector thinks about FER and FR, the go-to modality is the visible spectrum usually encoded as RGB. RGB images have dominated the area of FER, indicative through a variety of well known facial databases used in AI (i.e. CK+ FER 2013, FERET, EmotioNet, RECOLA, Affectiva-MIT Facial Expression Dataset, NovaEmotions, MultiPIE, McMaster Shoulder Pain, AffectNet, Aff-Wild2, the Japanese Female Facial Facial Expression database, and CASME II for microexpressions). Any internet search will reveal dozens of RGB facial image databases easily accessible and downloadable, such as the Top 15 list of facial recognition databases on Kaggle which are RGB images. In contrast we identified 14 thermal FER datasets in Table 1 whose numbers have increased since 2018 possibly due to the decreasing cost of thermal cameras and the easier ability to purchase them online.
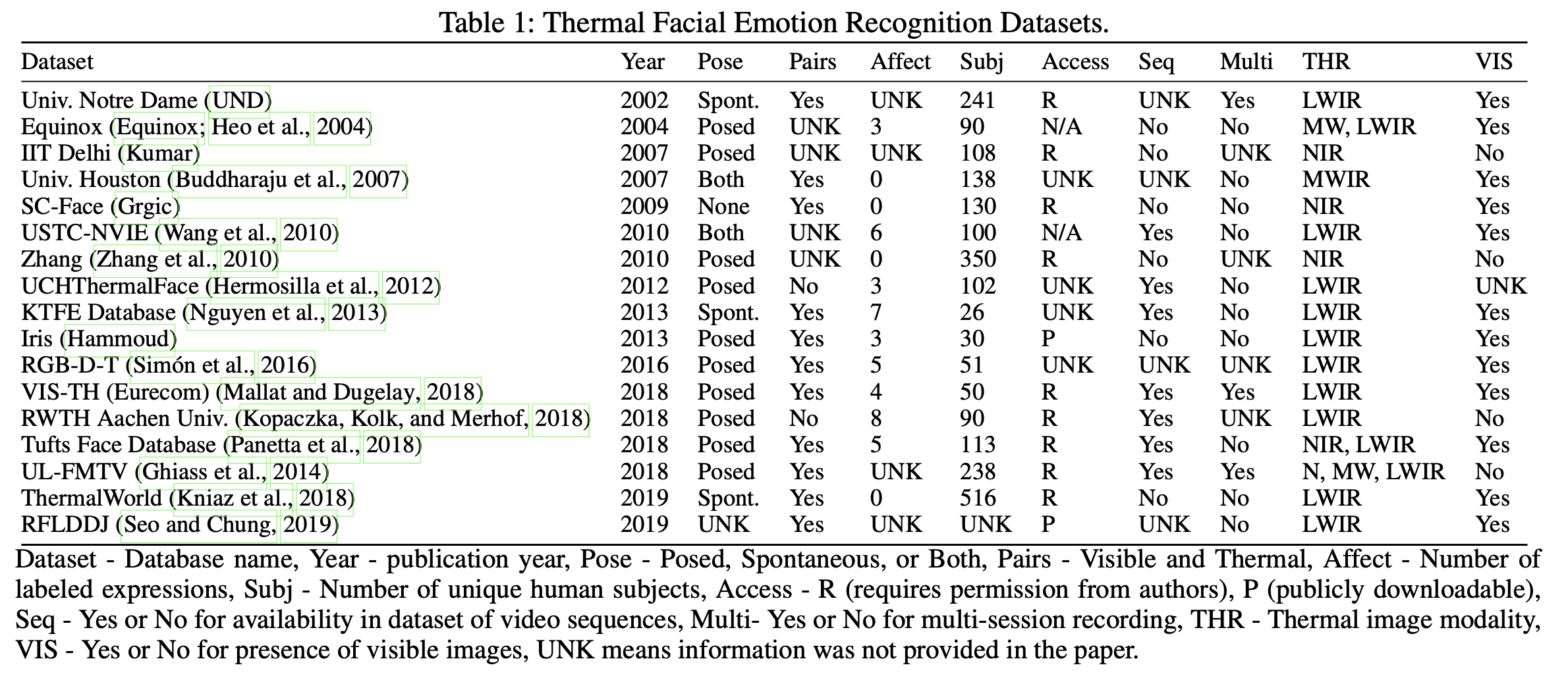
We believe that long-wave Infrared Radiation (LWIR) used alone, as a data source for FER, may be able to provide some form of anonymity for healthcare applications to minimize racial, ethnic, and potentially gender bias, when compared to RGB for FER. Through its low, grey-scale resolution 2 and reliance on temperature vectors driven by underlying vasculature (Ioannou, Gallese, and Merla, 2014), rather than superficial skin tone, texture, and pigmentation, thermal imagery can be more challenging to easily identify individuals. But there still remains a variety of issues to preserve privacy. For example, anonymity may not be possible if thermal FER is combined with the machine learning task of FR, especially since thermal FR is well researched with multiple methods proposed to detect and recognize individuals. The concept of separating FR from other tasks is not uncommon. Van Natta et al. (2020), question whether during Covid-19 temperature monitoring, there is even a need to conduct FR given how the overall purpose is to identify infection as opposed to identity. It is important to caution, that although thermal FR is more challenging than the visible domain, it is feasible to use thermal imagery as a “soft” biometric due to its invariance under lighting and pose (Reid et al., 2013; Friedrich and Yeshurun, 2002). For example, superficial vascular net- works are unique to each person’s face as proposed by Buddharaju et al. (2007), and can be extracted through methods like anisotropic diffusion to identify minutiae points akin to fingerprints as shown in Figure 3. Further, combining RGB with thermal can increase recognition accuracy. For example, Nguyen and Park (2016) used a combination of thermal and visible full body images for gender detection, finding that their proposed method of score-level fusion (training two separate SVM classifiers) combining thermal and visible led to a decrease in error of 14.672 equal error rate (EER) when compared to using thermal only (19.583 EER) and visible only (16.540 EER).
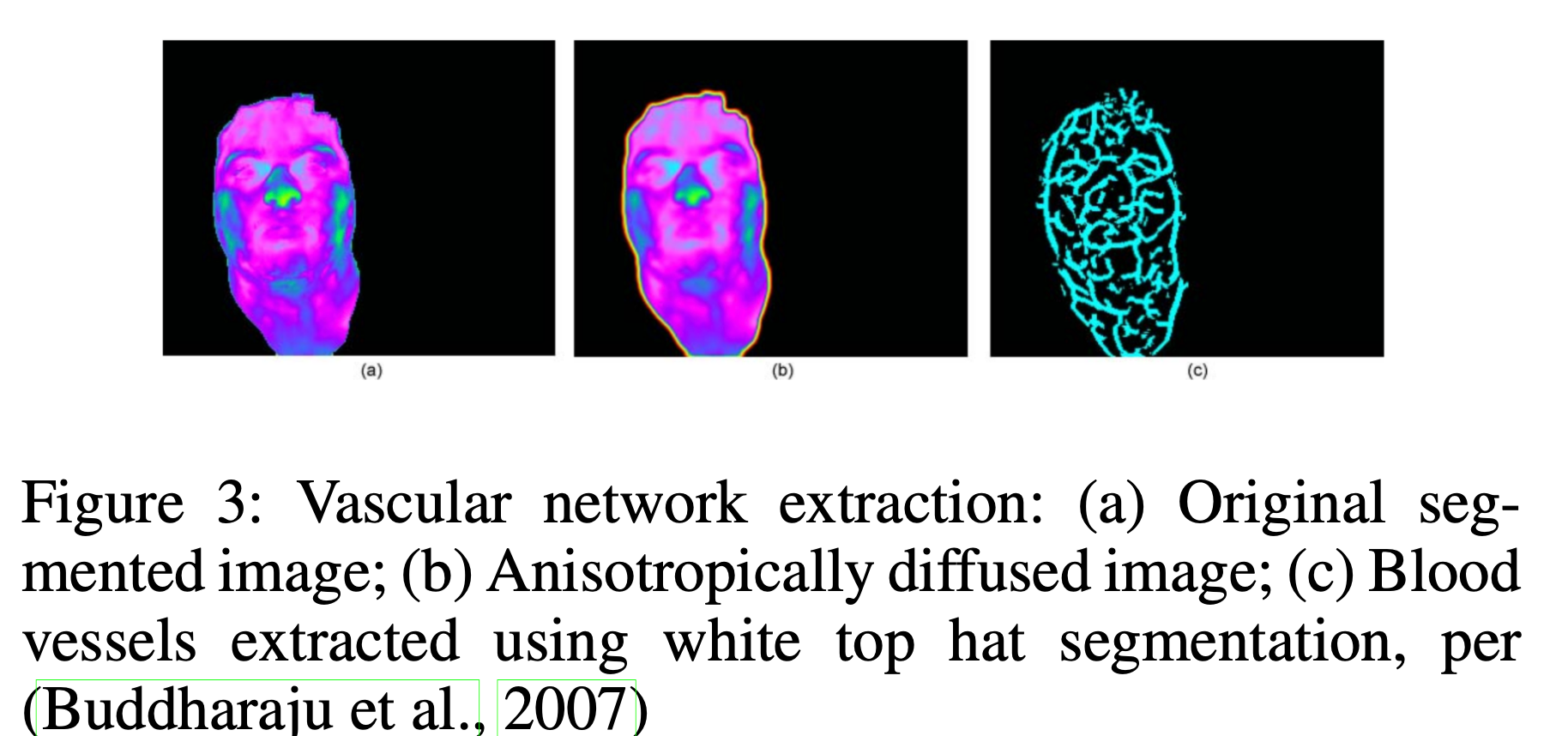
In addition, there has been research in the computer and electrical engineering fields to develop sensor-level privacy for thermal sensors in situations where people need to be sensed and tracked, but not identified. Work by Pittaluga, Zivkovic, and Koppal (2016) demonstrated different techniques to include digitization that masks human temperatures measurements thereby obscuring any ability to detect faces shown in Figure 4, manipulating the sensor noise parameters as the thermal image is being generated, and algorithms to under or overexpose specific pixels that are designated as “no capture” zones. Still in research, these techniques require different levels of hardware and firmware up- grades based on the thermal sensor.
Thermal imagery has additional technical advantages including how it is (1) invariant to lighting conditions un- like RGB, allowing the detection of physiological response (heat) to occur in low light or total darkness; (2) is a reliable and accurate correlation to standard physiological measures like respiration and heart rate; (3) is non-invasive i.e., requiring no skin contact whatsoever, making it convenient and non-intrusive and potentially relevant for non- communicative persons; (4) resistant to intentional deceit since physiological responses cannot be faked, whereas visible facial expressions can be controlled; and (5) is able to reveal facial disguises (i.e. wigs, masks) since these mate- rials have high reflectivity and display as the brightest on thermograms compared to human skin which is among the darkest objects with low reflectivity (Pavlidis and Symosek, 2000).
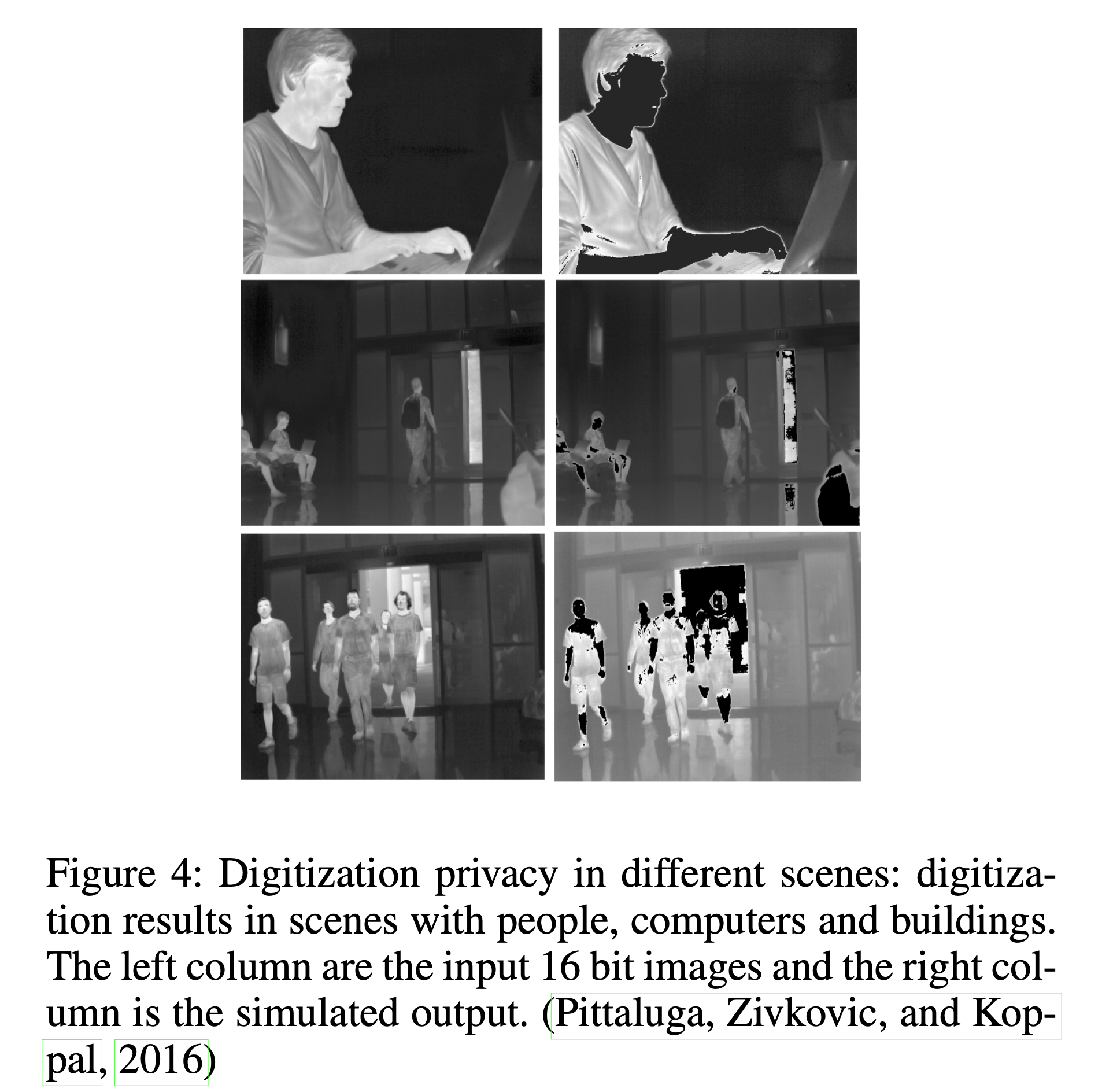
In addition, thermal imagery offers physiological signals of social interactions from person to person. In terms of deceit detection, it is valuable to note that RGB images can also be used to detect microexpressions using databases like CASME II. Microexpressions are genuine, quick facial movements that may be uncontrollable or unnoticeable by the individual, and therefore have been studied as an indication of deception (Yan et al., 2014). The RGB images used for studying microexpressions, however, are different than standard RGB FR datasets. They consist of video sequences captured using spontaneous natural elicitation, captured at a high frame rate of 200 fps, and labeled with facial action units (FAUs) which are encoded combination of facial movements based on Paul Ekman’s Facial Action Coding System (FACS) (Ekman, 1999).
Physiology and Thermal FER
A brief explanation of thermal radiation helps to understand how facial skin acts as a radiating surface. Thermal radiation is emitted by all objects above absolute zero (-273.15 ◦C). Human skin is estimated at 0.98 to 0.99 ε (Yoshitomi et al., 2000). The principal of thermal image generation is well understood by the Stefan-Boltzmann law that states total emit- ted radiation over time by a black body is proportional to T 4 where T is temperature in Kelvins: W = εσT 4 where W is radiant emittance (W/cm2), ε is emissivity, σ is the Stefan- Boltzmann constant (5.6705 · 10−12W/cm2K4), and T is Temperature (K).
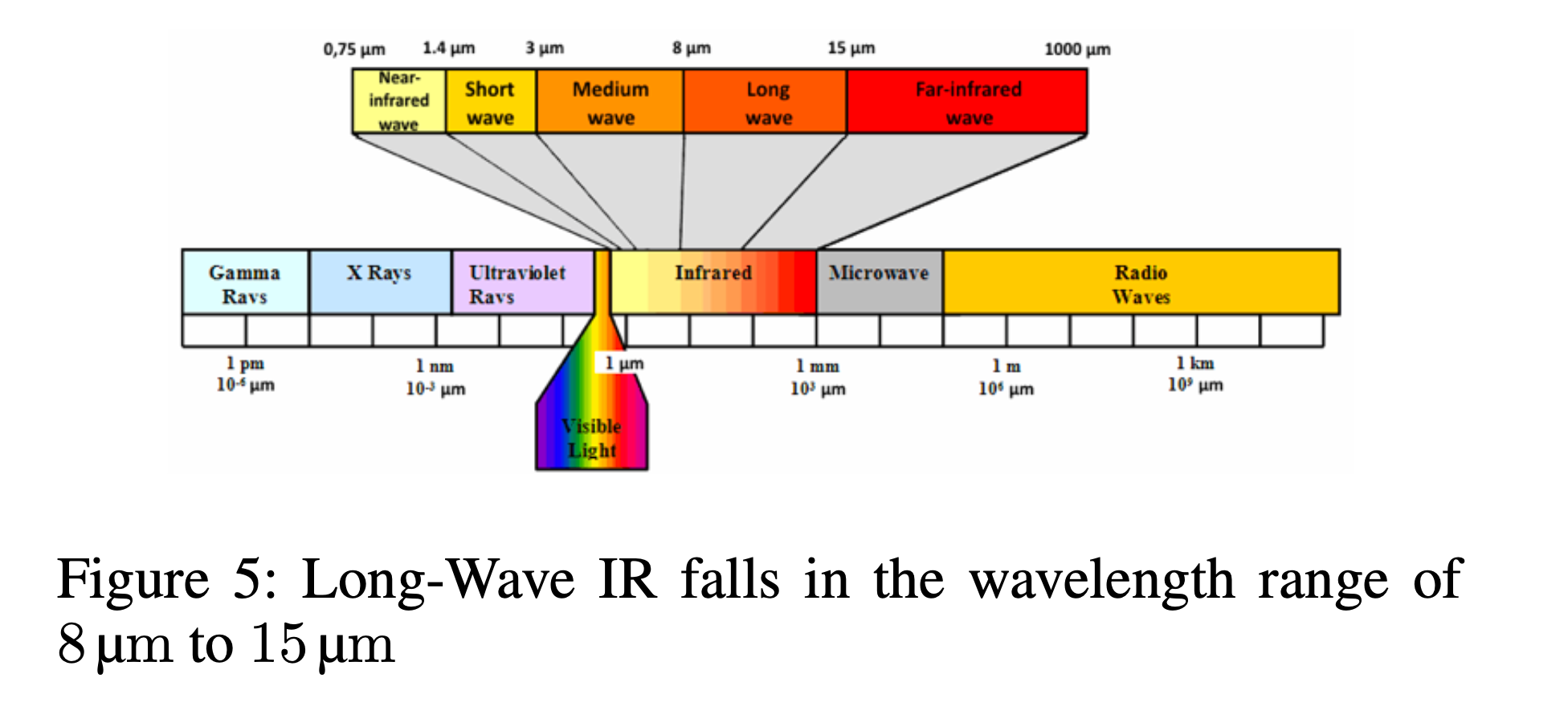
A black body is an object that absorbs all electromagnetic radiation it comes in contact with. No electromagnetic radiation passes through the black body and none is reflected. Since no visible light is reflected or transmitted, the object looks black upon visualization from thermal imagery, when it is cold. Thermal sensors respond to infrared radiation (IR) and produce visualizations of surface temperature. Because LWIR operates in a sub-band of the electromagnetic spectrum per Figure 5 it is invariant to illuminating conditions meaning that it can operate in low light to complete darkness. By imaging temperature variations to emotionally induced stimuli such as videos or pictures, thermograms reveal genuine responses to social situations. This occurs through activation of the autonomic nervous system (ANS) where emotional arousal leads to a perfusion of blood vessels innervated at the surface of the skin (Ioannou, Gallese, and Merla, 2014).
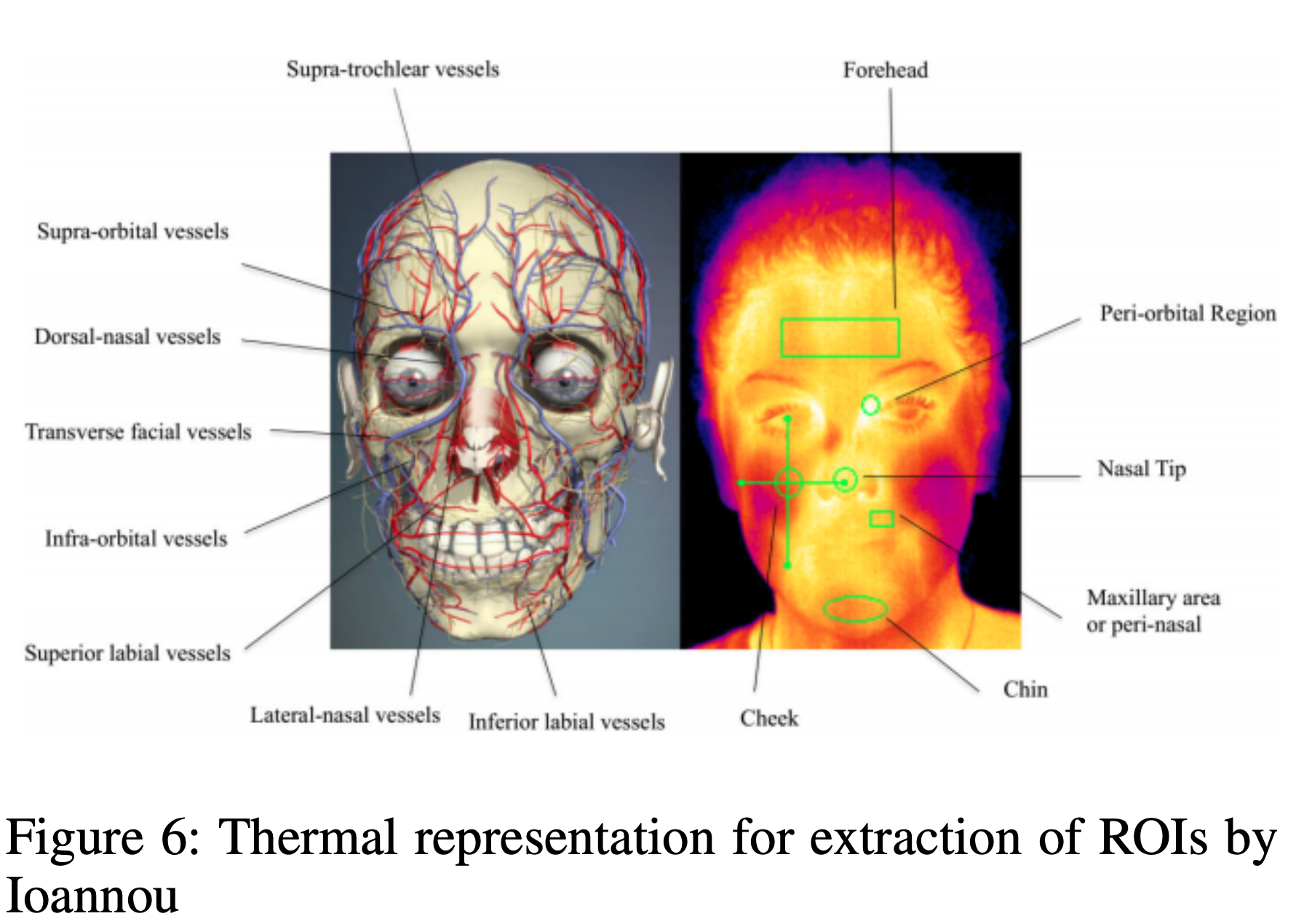
These images are called thermograms and are the data captured in thermal FER datasets, with labels based of the emotional response elicited (i.e. happiness, disgust, sadness, deceit, stress, etc.). Although today’s need for a touch-less system are paramount, the concept of using thermograms for contact-less physiological monitoring is not new and rooted in the intersection of physiological research (Selinger 2016;Buddharaju 2007;Pavlidis 2000; Ionnou 2014) and affective computing (Wilder 1996;Yoshitomi 2000;Goulart 2019). These include applications for FER where different emotions are detected from thermal facial images alone, in addition to person re-identification on thermal imagery, for FR. Since 1996 (Wilder et al., 1996) there have been numerous studies evaluating how thermograms correlate with vital measures. In 2007, Pavlidis (Pavlidis et al., 2007) demonstrated that thermal imagery is a reliable measure to assess emotional arousal where different regions of the face (zygomaticus, frontal, orbital, buccal, oral, nasal) correlate with different emotional responses. Thermal imagery also visualizes the physiology of perspiration (Pavlidis et al., 2012; Ebisch et al., 2012), cutaneous and subcutaneous temperature variations (Hahn et al., 2012; Merla et al., 2004), blood flow (Puri et al., 2005), cardiac pulse (Garbey et al., 2007), and metabolic breathing patterns (Pavlidis et al., 2012) and has been used to monitor heat stress and exertion (Bourlai et al., 2012). The reliability of thermal temperature readings have been repeatedly shown to be consistent and correlate accurately with gold standard physiological measures of electrocardiography (ECG), piezoelectric thorax stripe for breathing monitoring, nasal thermistors, skin conductance, or galvanic skin response (GSR) (Pavlidis et al., 2007; Sonkusare et al., 2019).
We can even observe these changes with the naked eye, such as embarrassment causing a person to blush (Sonkusare et al., 2019), or fear leading to pallor (Kosonogov et al., 2017). Merla (Merla, 2014) offered a survey of thermal studies in psychophysiology from 1990 to 2013, demonstrating a series of emotional responses detected on thermal imagery such as startle response, fear of pain, lie detection, mental workload, empathy, and guilt. These responses occur in different regions of the face, or ROIs. Salazar-Lopez found high arousal images elicited temperature increases on the tip of the nose (Salazar-Lopez et al., 2015). Kosnogov (Kosonogov et al., 2017) found that more arousing an image, the faster and greater the thermal response on the tip of the nose. He speculated that the speed and magnitude of these thermal responses were linked to autonomic adjustments normal to emotional situations. Zhu (Zhu, Tsiamyrtzis, and Pavlidis, 2007) found that deception was detected through increased forehead temperature and Puri (Puri et al., 2005) found the forehead to be correlated with stress. Social responses based on one-on-one personal contact can also be observed. For example, Ebisch (Ebisch et al., 2012) found “affective synchronization” of facial thermal responses between mother and child, where distress temperatures at the tip of the nose were mimicked by the mother as she watched her child in distress. Fernandez (Fernandez-Cuevasetal.,2015) summarizes analysis by Ioannou, Gallese, and Merla (2014) de- scribing whether temperature increases, decreases, or stays the same based on different emotions and ROIs provided in Figure 7.
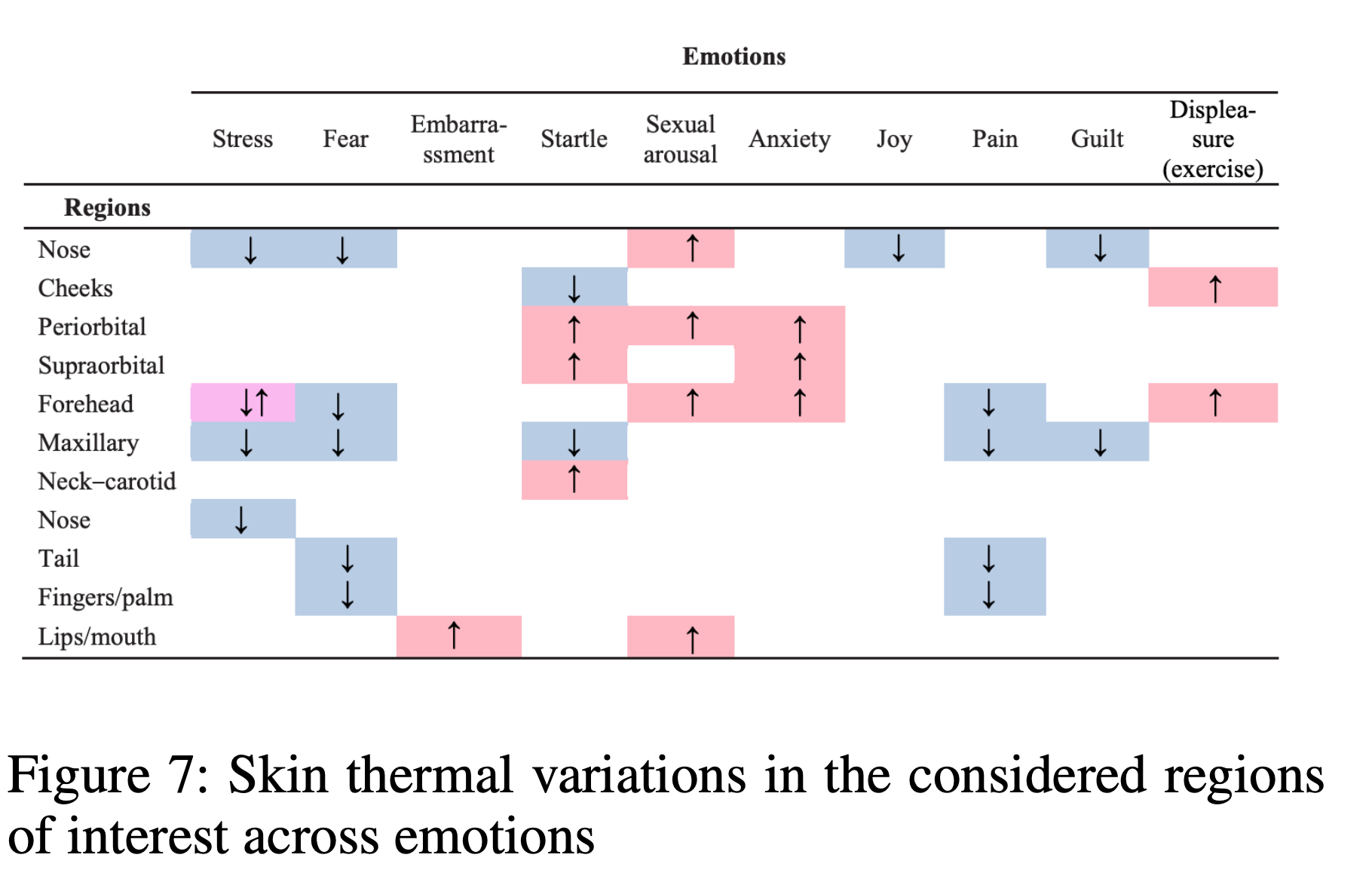