Faster, more Pythonic
I've been working on a paper analyzing Covid-19 tweets. I've finally hit a wall where I've successfully generated a variety of topic models. However, now I have to assign/predict the topics to each of my tweets. We're talking dozens of millions.
I hit a wall on my i9 if I try to run it against the entire dozen-million corpus. So, I decided to split it into batches of 250,000 at a time. Good idea, but still was pretty slow. I tried multiprocessing using the multiprocess library. I was overjoyed that it worked!
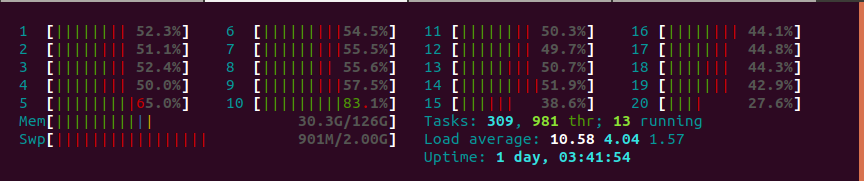
Oh wait, no, it didn't.
After pacing around and checking my watch a few times, this entire process actually took me longer than doing this serially. With one CPU it took about 31 minutes. With all 20, it took well, I stopped it at 50 minutes.
I can't wait this long. After some research, it seems like multiprocessing/threading is not the answer here. The answer is writing more Pythonic, compact code. So what is the difference between this function def predictions(), and def predictions_faster()?
def predictions(my_corpus, set_number, mode):
"""
Args:
my_corpus = gensim corpus
set_number = the corpus split (i.e. 1, 2, 3, etc.)
mode = train or test (str)
Returns:
list of labels for this corpus, you need to append this with the
previous set
"""
labels = []
for i in range(0,len(my_corpus)):
#get the corpus first and its topic
#topics are list of tuples [(2, 0.23769332), (13, 0.4979352),
(18,0.18703553)]
topics = best_lda.get_document_topics(my_corpus[i])
temp = []
for i in range (0, len(topics)):
#iterate through the probability value for each tuple
#like (2, 0.23769332)
probs = topics[i][1]
temp.append(probs) #put them all in a temp list
if len(set(temp)) == 1: #if uniform
#for exa: (0.5, 0.5, 0.5, 0.5)
labels.append(100)
elif len(set(temp)) != 1:
#get the max topic based on its prob
res = sorted(topics, key = lambda i: i[1], reverse = True)
[0][0]
labels.append(res) #get the topic at its max
with open('data/labels_{}_{}.pkl'.format(str(set_number),
str(mode)), 'wb') as f:
pickle.dump(labels, f)
return labels
A more Pythonic way = A faster way
Take a look at Python Speed Performance Tips, one of the improvements they suggest is to stop using for loops and use Pythonic list enumeration. Shame on me.
So, below we have a faster process just by enumerating a list instead of saving it to a for loop. Specifically getting rid of this hideous loop:
temp = []
for i in range (0, len(topics)):
probs = topics[i][1]
temp.append(probs)
with this:
temp = [item[1] for item in topics]
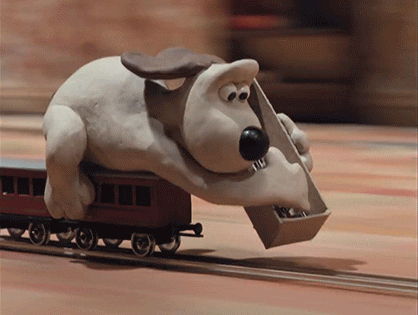
def predictions_faster(my_corpus, set_number, mode):
labels = []
for i in range(0,len(my_corpus)):
topics = best_lda.get_document_topics(my_corpus[i])
temp = [item[1] for item in topics] #stores all the probabilities
if len(set(temp)) == 1:
labels.append(100)
else:
res = sorted(topics,
key = lambda i: i[1],
reverse = True)[0][0]
labels.append(res)
with open('data/labels_{}_{}.pkl'.format(str(set_number), str(mode)),
'wb') as f:
pickle.dump(labels, f)
return labels
Input: A list of tuples of topics for each tokenized list of documents. Here showing the first five.
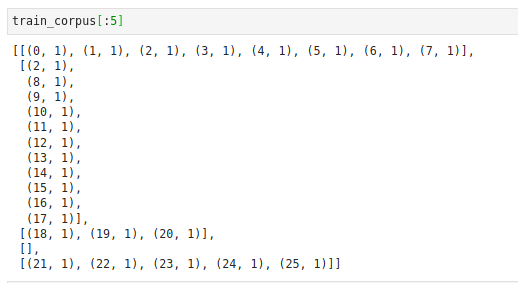
For any single item in the corpus, retrieve the predicted topics and its probability. Return the top item. But we have a problem with some lists that have all similar or uniform probabilities that automatically default to 0. We don't want to default to 0, we want to tag those a different label '100'.
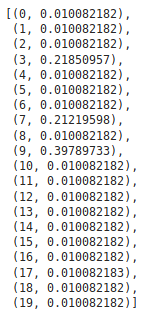
Output:
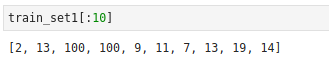
Time
batch of 250,000 tuples from a list:
- CPU times: user 39.6 s, sys: 11.4 ms, total: 39.6 s Wall time: 39.6 s
batch of 1,00,000 tuples from a list:
- CPU times: user 2min 35s, sys: 23.2 ms, total: 2min 35s Wall time: 2min 35s
batch of 2,500,000 tuples from a list:
- CPU times: user 5min 12s, sys: 47.5 ms, total: 5min 12s Wall time: 5min 12s