Amazon Food Reviews, Part V
In this final task, my goal is to predict the Amazon score (1 - 5) based on the reviews - a multiclass text classification problem. This post discusses the use of five pipeline models using scikit learn. The next post will introduce using Keras for building a sequential model for deep learning.
Scikit Learn
First, let's apply a typical test/train split:
from sklearn.cross_validation import KFold, train_test_split
from sklearn.cross_validation import cross_val_score
from scipy.stats import sem
from sklearn.feature_extraction.text import CountVectorizer
from sklearn.feature_extraction.text import TfidfTransformer
from sklearn.feature_extraction.text import TfidfVectorizer
from sklearn.feature_extraction.text import HashingVectorizer
from sklearn.naive_bayes import MultinomialNB
from sklearn.svm import LinearSVC
from sklearn.linear_model import LogisticRegression
from sklearn.pipeline import Pipeline
from sklearn import metrics
X, y = data2['text_cln'], data2['Score']
X_train, X_test, y_train, y_test = train_test_split(X, y,
test_size=0.2, random_state=0)
Next, let's set up our cross validation:
def evaluate_cross_validation(clf, X, y, K):
cv = KFold(len(y), K, shuffle=True, random_state=0)
scores = cross_val_score(clf, X, y, cv=cv)
print(scores)
print("Mean score:{0:.3f}(+/-{1:.3f})".format(np.mean(scores),sem(scores)))
Next, we create a 5 different pipelines to test out 5 different classifiers:
Creating multiple models through pipelines:
clf_1 = Pipeline([('vect', CountVectorizer(decode_error='ignore', strip_accents='unicode', stop_words='english')),
('clf', MultinomialNB()),
])
clf_2 = Pipeline([
('vect', HashingVectorizer(decode_error='ignore', strip_accents='unicode', stop_words='english', non_negative=True)),
('clf', MultinomialNB()),
])
clf_3 = Pipeline([
('vect', TfidfVectorizer(decode_error='ignore', strip_accents='unicode', stop_words='english')),
('clf', MultinomialNB(alpha=.01)),
])
clf_4 = Pipeline([
('vect', TfidfVectorizer(decode_error='ignore', strip_accents='unicode', stop_words='english')),
('clf', LogisticRegression()),
])
clf_5 = Pipeline([
('vect', TfidfVectorizer(decode_error='ignore', strip_accents='unicode', stop_words='english')),
('clf', LinearSVC()),
])
#Evaluate each model using the K-fold cross-validation with 5 folds
clfs = [clf_1, clf_2, clf_3, clf_4, clf_5]
for clf in clfs:
evaluate_cross_validation(clf, data2['text_cln'],
data2['Score'], 5)
Now, after we run evaluate each pipeline, we get an accuracy score for each of the five folds and a mean accuracy:
clf1 [ 0.70503382 0.70498984 0.70557036 0.70416304 0.70628903] Mean score:0.705(+/-0.000)clf2
[ 0.63822994 0.63876648 0.63848502 0.64095663 0.63752309]
Mean score:0.639(+/-0.001)
clf3
[ 0.70141876 0.70185855 0.70214881 0.70458524 0.7019087 ]
Mean score:0.702(+/-0.001)
clf4
[ 0.74205522 0.742926 0.7429348 0.74464118 0.74180667]
Mean score:0.743(+/-0.000)
clf5
[ 0.75884635 0.7610277 0.76046477 0.7631123 0.75872988]
Mean score:0.760(+/-0.001)
It's time to evaluate our models:
def train_and_evaluate(clf, X_train, X_test, y_train, y_test):
clf.fit(X_train, y_train)
print("Accuracy on training set:")
print(clf.score(X_train, y_train))
print("Accuracy on testing set:")
print(clf.score(X_test, y_test))
y_pred = clf.predict(X_test)
print("Classification Report:")
print(metrics.classification_report(y_test, y_pred))
print("Confusion Matrix:")
print(metrics.confusion_matrix(y_test, y_pred))
Now we can review the accuracy, classification report, and confusion matrix for each clf:
clf1
train_and_evaluate(clf_1, X_train, X_test, y_train, y_test)
Accuracy on training set:
0.731330385278
Accuracy on testing set:
0.705033819739
Classification Report:
class precision recall f1-score support
1 0.56 0.64 0.59 10267
2 0.47 0.20 0.28 6185
3 0.43 0.28 0.34 8450
4 0.40 0.34 0.37 16229
5 0.81 0.89 0.84 72560
avg
/total 0.68 0.71 0.69 113691
Confusion Matrix:
[[ 6533 689 654 479 1912]
[ 1443 1226 968 787 1761]
[ 1089 296 2371 1804 2890]
[ 767 141 770 5573 8978]
[ 1883 235 797 5192 64453]]
clf2
train_and_evaluate(clf_2, X_train, X_test, y_train, y_test)
Accuracy on training set:
0.638943801497
Accuracy on testing set:
0.638229939045
Classification Report:
class precision recall f1-score support
1 1.00 0.00 0.00 10267
2 0.00 0.00 0.00 6185
3 0.00 0.00 0.00 8450
4 0.00 0.00 0.00 16229
5 0.64 1.00 0.78 72560
avg
/ total 0.50 0.64 0.50 113691
Confusion Matrix:
[[ 1 0 0 0 10266]
[ 0 0 0 0 6185]
[ 0 0 0 0 8450]
[ 0 0 0 0 16229]
[ 0 0 0 0 72560]]
clf3
train_and_evaluate(clf_3, X_train, X_test, y_train, y_test)
Accuracy on training set:
0.743461979097
Accuracy on testing set:
0.701418757861
Classification Report:
class precision recall f1-score support
1 0.74 0.44 0.55 10267
2 0.76 0.11 0.20 6185
3 0.67 0.12 0.20 8450
4 0.61 0.12 0.21 16229
5 0.70 0.99 0.82 72560
avg
/total 0.69 0.70 0.63 113691
Confusion Matrix:
[[ 4479 106 141 94 5447]
[ 637 708 144 190 4506]
[ 426 59 984 424 6557]
[ 231 28 103 2005 13862]
[ 313 31 93 554 71569]]
clf4
train_and_evaluate(clf_4, X_train, X_test, y_train, y_test)
Accuracy on training set:
0.761820992473
Accuracy on testing set:
0.742064015621
Classification Report:
class precision recall f1-score support
1 0.68 0.67 0.68 10267
2 0.60 0.19 0.29 6185
3 0.54 0.27 0.36 8450
4 0.53 0.24 0.33 16229
5 0.78 0.97 0.86 72560
avg
/total 0.71 0.74 0.70 113691
Confusion Matrix:
[[ 6911 336 304 240 2476]
[ 1520 1183 762 471 2249]
[ 791 284 2259 1239 3877]
[ 377 95 557 3926 11274]
[ 583 79 335 1476 70087]]
clf5
train_and_evaluate(clf_5, X_train, X_test, y_train, y_test)
Accuracy on training set:
0.814890393458
Accuracy on testing set:
0.758846346677
Classification Report:
class precision recall f1-score support
1 0.69 0.71 0.70 10267
2 0.62 0.29 0.40 6185
3 0.57 0.34 0.42 8450
4 0.57 0.30 0.39 16229
5 0.80 0.96 0.87 72560
avg
/total 0.73 0.76 0.73 113691
Confusion Matrix:
[[ 7305 391 336 284 1951]
[ 1452 1821 678 464 1770]
[ 790 401 2859 1168 3232]
[ 404 157 670 4891 10107]
[ 687 176 482 1817 69398]]
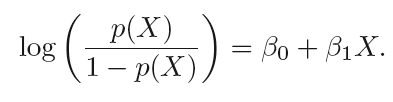
Ref:https://www.jvrb.org/past-issues/3.2006/760
Now, let's see how well clf_4 (logistic regression) and clf_5 (LinearSVC) predicts on a few real Amazon reviews. I wanted to actually use a few neat Amazon product review webscrapers out there, but realized that I needed to have my own AWS account to access the API and get a token. I guess that'll be on my next bucket list. Here were some resources for those of you interested:
Now, back to Cheetos. I chose just four reviews of varying star-ratings and wrote them into a tiny corpus:
corpus = [
"Perfect couldnt be any better it came in under 3 and a half days and it taste so good like wow and it feals like it has more then regular bags just amazing i recomend getting it",
"husband loves these but i can't take the orange fingers. still he loves them so....",
"They smelled and tasted like dog poop",
"Theyre are good. But youre paying 10 Dollars for a bag of chips."
]
Now, just like with the model pipelines, you need to vectorize this corpus:
tf_vectorizer = CountVectorizer(max_df=0.95, min_df=2,stop_words='english')
vect = tf_vectorizer.fit_transform(corpus)
LinearSVC doesn't offer a predict_probaba() method, although I did read here that "scikit-learn provides CalibratedClassifierCV which can be used to solve this problem: it allows to add probability output to LinearSVC or any other classifier which implements decision_function method". I tried running this as my fourth classifier, but it just about took forever (sorry, didn't time it) on my Mac Book Pro, so I just kinda gave up and decided to kick the tires on ye old logistic regression instead.
Prediction for the LinearSVC which had the highest training accuracy:
predicted = clf_5.predict(corpus)
print(predicted)
[5 5 1 4]
Prediction for the Logistic Regression model with the second highest training accuracy. Interesting to see here that it predicted the fourth review: "Theyre are good. But youre paying 10 Dollars for a bag of chips", as a 5-star not a 4-star.
predicted=clf_4.predict(corpus)
probas = clf_4.predict_proba(corpus)
print(predicted, probas)
[5 5 1 5]
[[ 3.75934195e-04 3.63309774e-03 2.19956217e-03 8.07333606e-02 9.13058045e-01]
[ 2.03279754e-04 1.85061482e-03 8.29297196e-03 3.92534317e-02 9.50399702e-01]
[ 4.43243634e-01 9.96319899e-02 1.78067717e-01 2.84542527e-02 2.50602406e-01]
[ 1.15504075e-01 9.87625810e-02 1.83887123e-01 2.57270762e-01 3.44575459e-01]]
In summary, here are some good next steps we could take for a follow on model:
- Use GridSearch to better tune the hyperparameters for Logistic Regression and LinearSVC
- Use the Confusion Matrix to identify the reviews that suffered from low recall (both models performed poorly with recall - The percent of true positives out of all positives)
- Potentially try a deep learning model...